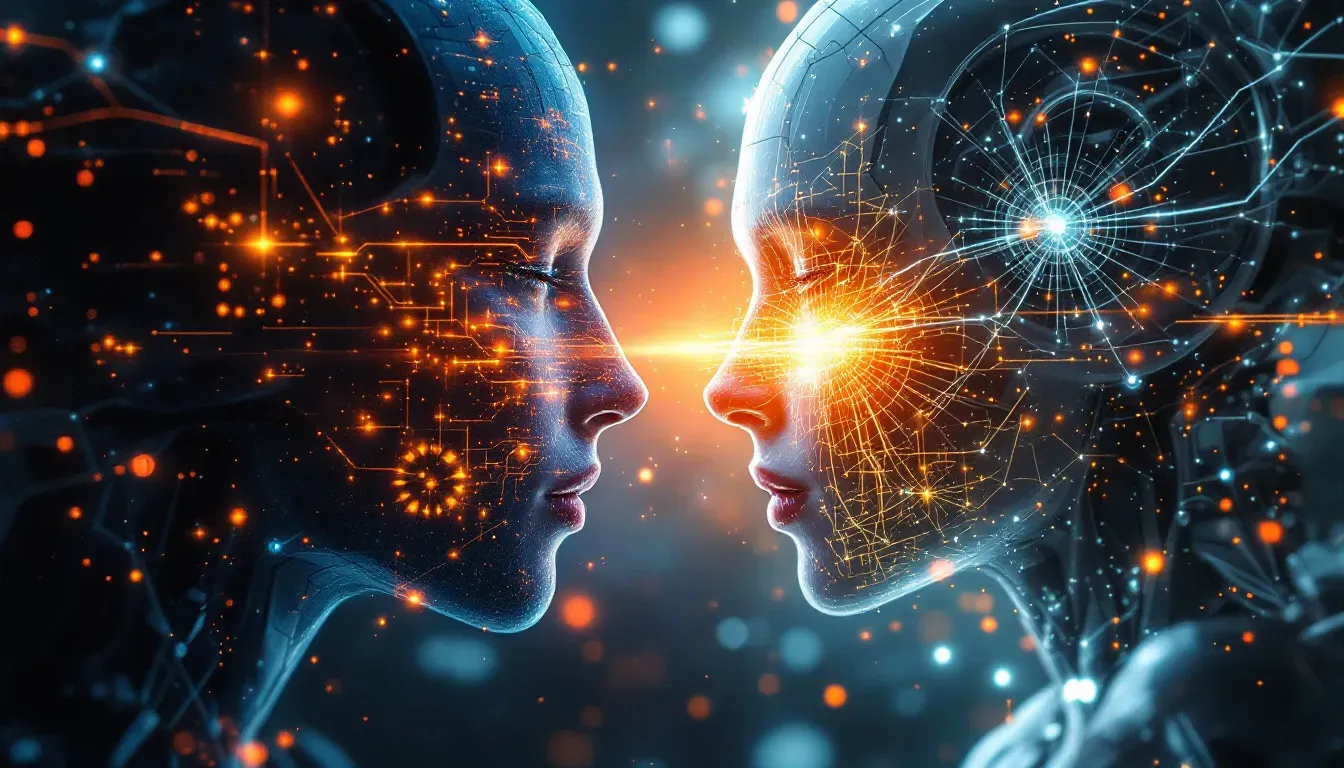
Ethical AI: Balancing Efficiency with Responsibility
Ethical AI is no longer an abstract concept but an everyday reality that demands deliberate attention and action. From advanced chatbots that instantly produce human-like text to image generators capable of synthesizing photorealistic scenes, generative AI’s capabilities are transforming the ways we live and work. Yet behind these gains in efficiency and creativity loom significant ethical challenges—bias, opacity, accountability, and the potential misuse of AI-generated content, among others.
This tension between efficiency and responsibility is particularly important for small and medium-sized businesses (SMBs). While advanced AI has historically been dominated by large enterprises, recent developments have brought these technologies within reach of smaller organizations. Whether you’re a managed service provider (MSP) automating IT support, a boutique law firm drafting documents more quickly, or a healthcare clinic looking to streamline administrative work with AI-driven triage, you will confront both the benefits and risks of generative AI.
A Short History of AI Ethics
From Science Fiction to Boardroom Priority
The conversation around AI ethics dates back decades, famously foreshadowed by Isaac Asimov’s fictional Three Laws of Robotics in the mid-20th century. But the ethical dimension of AI truly took shape in the public sphere once real-world AI applications began making headlines for their failures and biases.
A key turning point was Microsoft’s Tay chatbot (2016), which began spewing hateful content online due to a lack of safeguards. Incidents like this demonstrated how rapidly an AI system could cause harm if it was not carefully monitored. These early missteps prompted researchers, NGOs, and corporations to advocate for formal AI ethical frameworks, culminating in principles such as the Asilomar AI Principles (2017), the EU’s Ethics Guidelines for Trustworthy AI (2019), and later the UNESCO Recommendation on AI Ethics (2021).
Regulatory Progress
By the early 2020s, regulation became a focal point. The European Commission’s proposed AI Act took a risk-based approach, classifying AI systems according to their potential for harm, with specific requirements for “high-risk” systems (e.g., used in recruiting, medical devices, or transportation). In the United States, the AI regulatory environment became a patchwork of sector-specific rules, enforcement actions from the Federal Trade Commission (FTC) or Equal Employment Opportunity Commission (EEOC), and guidance from the White House’s Blueprint for an AI Bill of Rights.
For businesses—large or small—this growing patchwork of guidelines and regulations signaled that ignoring AI ethics was no longer an option. The question shifted from, “Should we consider ethics?” to “How do we practically integrate ethics into our AI systems?”
Ethical Challenges of Generative AI
Generative AI—capable of producing text, images, code, and other forms of content—has introduced new hurdles that go beyond the more traditional analytics-oriented AI of the past. While these technologies open exciting possibilities, four key challenges loom large:
Transparency and Explainability
Generative models typically function as “black boxes,” producing outputs based on vast amounts of training data without the ability to explain clearly how they reached a certain conclusion or why a piece of text or image looks the way it does. This opacity complicates trust, oversight, and regulatory compliance.
Bias and Fairness
AI systems learn from historical data that may carry biases related to race, gender, or culture. For instance, a text-generation tool could inadvertently produce stereotypical or discriminatory content, damaging a company’s reputation and potentially triggering lawsuits if used in a customer-facing environment.
Accountability
When something goes wrong—whether it’s misinformation, a biased hiring decision, or a harmful product recommendation—who is held responsible? Legal frameworks are still evolving, but current business best practices demand human oversight. SMBs, in particular, can’t afford the legal exposure that comes from blindly trusting AI outputs.
Misuse and Malicious Use
The power to create highly persuasive text, images, or videos at scale can be exploited for misinformation, harassment, or fraud. Deepfakes and synthetic media are already proliferating, testing the resilience of businesses and society alike. Malicious actors can leverage generative AI to produce more convincing phishing emails or financial scams.
Why It Matters for SMBs: Risks and Opportunities
Leveling the Playing Field
On the upside, generative AI can act as a force multiplier for SMBs. By automating complex tasks—ranging from creating social media marketing copy to drafting legal contracts—these technologies enable smaller organizations to compete with larger enterprises. Surveys from 2024–2025 revealed that SMBs adopting generative AI often cited increased productivity and the ability to offer services previously out of their reach.
For example, a small marketing agency can use AI-driven content generation to produce client-facing materials quickly without hiring multiple full-time writers. Similarly, a single IT professional at a small managed service provider might automate customer support queries, handling higher volumes of tickets and delivering faster response times.
Operational Risks
Nevertheless, with new power comes new risks:
• Hallucinations and Errors: AI models occasionally “hallucinate,” presenting incorrect information as fact. SMBs lacking robust quality-assurance processes could, for instance, forward erroneous advice to a client or misquote legal references.
• Bias and Discrimination: If a local insurance agency uses generative AI for claim analysis but the model is trained on biased data, entire demographics could experience unfair treatment, exposing the agency to legal and ethical repercussions.
• Privacy and Data Protection: Healthcare clinics or small educational institutions often handle sensitive personal data, and inadvertently feeding such data into public AI services can violate regulations like the EU’s General Data Protection Regulation (GDPR) or HIPAA in the U.S.
• Misuse and Reputation Management: In the event of AI misuse by an employee—such as providing misleading product information through a chatbot—an SMB may quickly face tarnished brand reputation and potential legal claims.
Industry Snapshots
1. Managed Service Providers (MSPs):
MSPs can embed generative AI in helpdesk operations to automate routine queries. Done responsibly, this raises productivity and client satisfaction. Done poorly—without stringent data privacy or error-correction loops—it can lead to client mistrust and potentially serious data leaks.
2. Legal Services:
Small law firms can offload the drudgery of contract drafting, but still must meticulously review AI-generated content. AI “hallucinations” can be devastating if they introduce fake case law or incorrect citations, risking sanctions or malpractice claims.
3. Insurance:
Streamlining claim evaluations through AI can speed up processing. However, embedded biases in underwriting or claims denial can prompt regulatory probes and lawsuits, particularly if specific populations are adversely affected at higher rates.
4. Higher Education:
College admissions, automated grading, or student advising may become more efficient, but institutions have to protect academic integrity. Students might turn to AI to cheat, while the institution must decide how—and whether—to incorporate AI as a learning tool without promoting dishonest practices.
5. Healthcare:
Generative AI can draft patient summaries and streamline triage. The downside is the high stakes of incorrect or “hallucinated” medical advice, especially if a small clinic relies heavily on AI support and lacks thorough human oversight.
Across these industries, the throughline is clear: generative AI can be a catalyst for growth and innovation, but it demands a strong ethical backbone to ensure its benefits outweigh its risks.
Future Outlook: The Next Decade
Short Term (3 Years)
Over the next three years, the widespread implementation of the EU AI Act and similar guidelines globally will standardize many ethical requirements. SMBs can expect:
• Mandatory AI Audits: More frequent and detailed audits to check for fairness and bias, as well as compliance with regulations.
• Certification and Labeling: “AI ethics certifications” or standardized “model cards” may become prevalent, helping both businesses and consumers understand how an AI system was trained and tested.
• Advanced Monitoring Tools: Businesses will use real-time dashboards and software solutions to detect drift or bias in AI systems, making it easier to intervene before harm escalates.
Mid Term (5 Years)
By 2030, ethical AI is likely to be so entrenched in everyday practice that any organization deploying it haphazardly stands out as an outlier. Expect:
• Widespread Explainability Methods: Emerging breakthroughs will offer more user-friendly ways to interpret a model’s outputs.
• Integrated Safeguards: Generative AI platforms may come with built-in fact-checking subroutines to reduce hallucinations and misinformation, offering a new level of reliability.
• Culture of Accountability: Roles such as “Responsible AI Officer” or ethics committees within SMBs may become commonplace, and companies will increasingly market their “ethical AI compliance” as a competitive advantage.
Long Term (10 Years)
Looking a decade ahead, we might see:
• International AI Regulatory Bodies: Similar to how nuclear or environmental concerns are managed across borders, AI might be overseen by international agreements or specialized agencies.
• AI Co-Governance by AI: Advanced meta-AI systems could monitor other AI processes for bias, drift, or harmful behavior.
• Deep Societal Impact and Debates: If AI capabilities approach near-human or superhuman levels in many domains, ethical considerations will expand to include labor displacement, AI “rights” or legal status, and sustainable energy usage for massive AI computations.
Across all time horizons, one message resonates: the future belongs to organizations that seamlessly merge efficiency with accountability. Ethical AI is evolving from a voluntary best practice to a business imperative.
Ethical AI in Humanoid Robots
One of the most fascinating extensions of generative AI lies in its embodiment within humanoid robots. While such devices can bring futuristic convenience—like lifelike customer service or healthcare assistance—the ethical stakes become more acute:
• Physical Safety: A mistake by a purely digital AI might lead to misinformation; a mistake by a physically mobile robot could cause actual harm or property damage.
• Emotional Manipulation: Anthropomorphized robots may engender high levels of trust or emotional attachment in users. Businesses must be mindful not to exploit this trust—especially in vulnerable populations such as children or the elderly.
• Regulatory Approaches: Governments will likely introduce more stringent rules for physically autonomous robots, including mandatory “kill switches,” logging requirements, and specialized certifications. Small clinics or campus security offices, for instance, will need to weigh the liability associated with using semi-autonomous guard robots.
With humanoid robots, accountability must be ironclad. Organizations cannot deflect blame onto a “rogue” AI robot; legally and ethically, ultimate responsibility rests squarely with the humans and businesses deploying these systems.
SMB Leadership in Ethical AI: How to Get Ahead
SMBs can—and should—take a leadership role in ethical AI adoption. By being transparent, proactive, and diligent, smaller organizations can differentiate themselves and foster greater trust among customers, regulators, and partners.
Key Recommendations
1. Develop an In-House AI Ethics Framework:
Even a short, well-considered document outlining how AI tools are chosen, validated, and monitored goes a long way. Make it clear who is accountable for AI decisions, how user data is protected, and what steps are taken to mitigate bias.
2. Train Your Team:
Provide basic AI literacy to every employee touching the AI lifecycle, from junior customer service reps to executive leadership. Encourage them to question AI outputs and report potential problems through a clearly defined channel.
3. Embed Ethics by Design:
Incorporate ethical considerations from the outset of any AI project. Ask how the training data was collected, how the model was tested for bias, and who will be harmed if the system fails. This approach mirrors Institutional Review Boards (IRBs) in academic research but scaled for business use.
4. Ensure Regulatory Compliance and Foresight:
Stay informed about emerging AI regulations in all the markets you serve—especially if you have clients or data in Europe, where the EU AI Act is poised to set global precedents. Voluntary compliance with recognized standards (e.g., ISO 42001 for AI management) can preempt future regulatory hurdles.
5. Practice Radical Transparency:
Let customers and partners know when AI is being used, how it’s being used, and what measures are in place to ensure ethical treatment of their data. Should errors or controversies arise, provide clear communication about how you plan to resolve the issue and improve.
6. Collaborate with Industry Peers:
Join local tech councils, AI ethics alliances, or cross-industry working groups. These collaborations can help you learn best practices, share solutions, and collectively shape more reasonable policies. By banding together, SMBs also ensure their needs are represented in policy discussions.
7. Monitor and Iterate Continuously:
Conduct regular reviews of AI performance, checking for any drift in accuracy or fairness. Update training data and refine models periodically to keep up with changing real-world conditions. Ethical AI is not a “set-and-forget” process; it requires ongoing vigilance.
Business Benefits of Ethical Leadership
Though it can require time, effort, and resources, leading with an ethical approach to AI offers tangible upsides:
• Stronger Brand Reputation: Consumers and partners gravitate toward companies that handle data responsibly and champion fairness, particularly when they perceive a potential risk to their own privacy or well-being.
• Lower Compliance Risks: Early adherence to AI best practices and upcoming regulations reduces the likelihood of costly legal battles or fines down the line.
• Enhanced Innovation: By systematically auditing AI systems, you can uncover gaps that lead to improvements in both accuracy and creativity. Meanwhile, a culture of open inquiry around AI ethics can spark broader organizational innovation.
Conclusion: Why Ethical AI Matters—and How SMBs Can Thrive
Generative AI stands among the most transformative technologies we’ve seen in decades, but it is also rife with ethical pitfalls that can quickly ensnare the unprepared. For SMBs, the stakes are perhaps even higher than for large enterprises. A single high-profile AI failure—whether it’s a biased hiring tool, a data privacy breach, or a deepfake-driven scandal—could tarnish an SMB’s reputation beyond repair.
Yet the other side of the coin is far more promising. By acting responsibly, SMBs can harness the same AI-driven innovations and stand out as ethical pioneers in their respective markets. The agility of smaller organizations can be an asset—leaders can pivot quickly to adopt new ethical standards, deploy rigorous training processes, and integrate advanced monitoring solutions.
Over the next decade, as regulations tighten and consumer expectations rise, “ethical AI” will move from being a desirable differentiator to a baseline requirement. Embracing that reality now allows SMBs to gain a competitive edge. Whether you’re automating customer support, drafting legal briefs, or installing a humanoid robot to greet visitors, the principles remain the same: be transparent, be accountable, and never let efficiency overshadow your commitment to human well-being and trust.
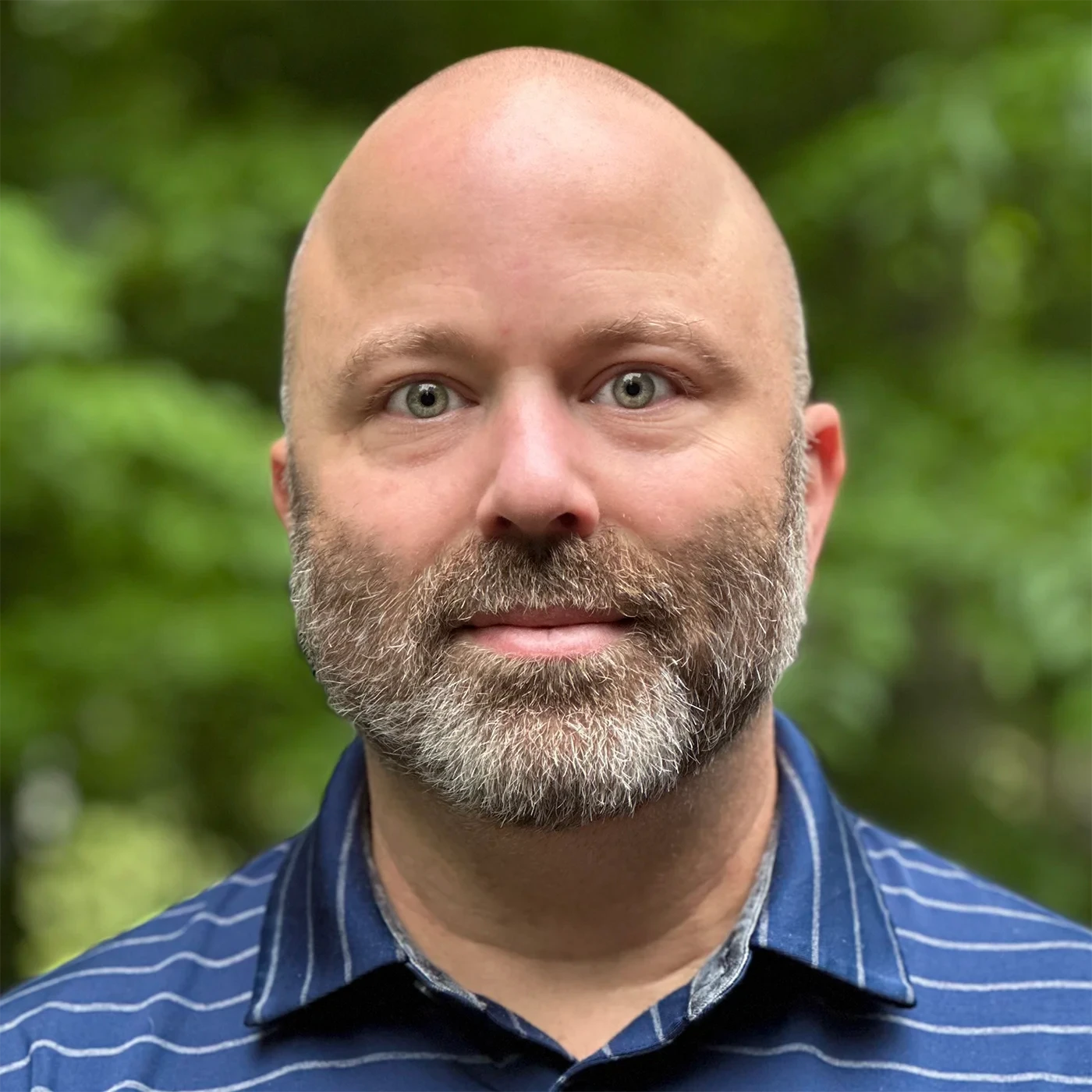